COVID-19 and Big Data
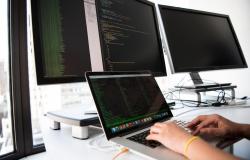
Nayef Al-Rodhan overviews some of the opportunities and worries about the use of big data to address pandemics.
The COVID19 pandemic has re-energized the interest in Big Data and in leveraging the power of predictive tools to help fight pandemics. That interest is understandable, seeing as this pandemic is taking place in times of ubiquitous digital data, a valuable resource, generated at a far higher speed compared to pandemics a decade or two ago (e.g. the SARS 2002-2003 outbreak).
Data and technology have already played a more meaningful role in managing this crisis. On March 23, the COVID-19 High Performance Computing Consortium was created, a public-private collaboration announced by the White House, which makes even NASA supercomputers available to execute complex computational research to help scientific progress. On April 20, the European Commission launched a Big data platform for COVID-19 research, where researchers can share and store DNA sequencing data and protein structures from pre-clinical research.
Bioinformatics, epidemiology and molecular modeling need huge computational capacity, but the use of Big Data is not limited to clinical settings – or for predictions on agriculture and the economy. Given the potential to use data from ‘real-world sources’ through apps or other digital records of personal movement, social media and personal medical records, Big Data has rapidly gained prominence as a possible solution to containing the spread of the virus. This comes, however, with significant risks for privacy despite the promise of anonymity, including in the UK, which launched a contact tracing app on 4 May, and in France, which rolled out a tracing app in early June.
(Big) Data and outbreaks
Digital health tools can speed up the process of identifying outbreaks by tracking real-time developments. Examples include the Google Flu Trends algorithm and the Influenzanet system created in Europe. There are numerous limitations to these approaches but, as a Nature study shows, in many instances, a smart wrangling of data can ‘buy the medical community extra days or weeks in which to act’.
The COVID19 pandemic stirred a more dynamic search for solutions with the help of Big Data, both for analyzing and forecasting the speed of contaminations, and the economic implications. Additionally, Big Data also received attention for potentially helping individuals with ‘personalized prediction’: using multiple sources of data, machine-learning models could measure an individual’s clinical risk of severe illness (e.g. probability of intensive care), and their risks to others.
In the early stages of the epidemic in China, the authorities installed thermal scanners in train stations, which permitted quick detection of suspected infections and testing. If the test came positive, the authorities would quickly notify all people that may have been in contact with that person. Taiwan also relied heavily on technology from early-on, aided by a system that integrates national health insurance and information on immigration and customs database.
Limitations and trade-offs
Relying on digital sources can be especially valuable during an outbreak, but it is important to understand the scope of these tools and maintain realistic expectations.
A sober assessment, both of capabilities and implications, is critical.
A first consideration is that data in itself – as extensive as datasets could be – can rarely capture the complexity of all social dynamics everywhere. For example, during the 2014-2016 Ebola epidemic, computational epidemiologists at Harvard used data obtained from phone users in West Africa to predict the spread of the virus based on the assumption that people’s movements were the main vector of transmission, whereas the spread happened largely through caring for the sick and during the funerals preparation. Using phone data for tracking people’s movements in the COVID 19 pandemic also risks missing important variables in understanding the spread of the virus. Additionally, conclusions may be distorted by differences in social media and cell phone penetration among populations, as well as by some environmental factors including high-rise buildings, which can distort location accuracy.
Furthermore, truly global forecasts are difficult. While in certain national contexts, as was the case in Taiwan, the use of big data yielded excellent results, a globally integrated approach is not yet feasible. This would require a standardization of sources of data across national systems, including information-sharing between hospitals and the public sector – a very difficult feat in most countries where data does not just flow easily from health establishments to the private sector, and global collaboration.
Finally, considerations about privacy must not be an afterthought. So far, it appears that apps rolled out in Western countries are premised on anonymous and aggregated data. In the European Union, the General Data Protection Regulation (GDPR) allows tracking for public health purposes, yet there is a consensus that privacy must be protected as much as possible, but that may not be the case in countries with limited accountability oversight mechanisms. It is critical not to sacrifice the protection of privacy, particularly when the benefits do not justify the costs, or when alternative data-based methods can be implemented instead.
The earliest responses to the pandemic were based on mathematical projections and computer simulations. Researchers at Imperial College, UK, used both agent-based and equation-based models and published the first report on March 16, which predicted likely numbers of deaths in the absence of any action, as well as other aspects such as critical care bed capacity. Information is loosely estimated at the beginning of a pandemic and simulations are not perfectly reliable, which requires constant updating and tweaking.
Big data is here to stay, and its utilization could be extremely useful in predicting and mitigating national and global cascading risks, like pandemics. Big data is also useful in guiding policy choices in general, but it is critical in all cases to address privacy concerns and guard against compromising civil liberties.
Prof. Nayef Al-Rodhan (@SustainHistory) is a Neuroscientist, Philosopher and Geostrategist. He is an Honorary Fellow at St Antony’s College, University of Oxford, and Senior Fellow and Head of the Geopolitics and Global Futures Programme at the Geneva Centre for Security Policy, Geneva, Switzerland. Through many innovative books and articles, he has made significant conceptual contributions to the application of the field of neurophilosophy to human nature, history, contemporary geopolitics, international relations, cultural studies, future studies, and war and peace.
Photo by Christina Morillo from Pexels